Time series analysis in demand planning
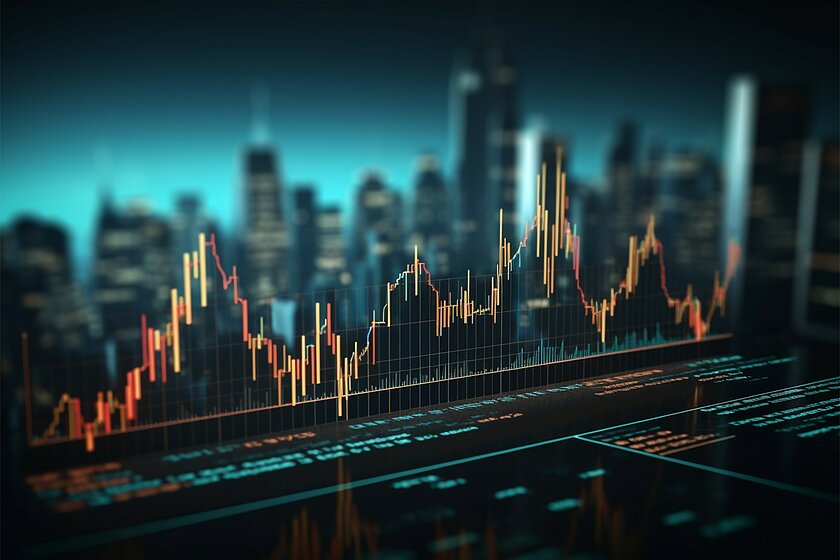
Overview
- Project Term
- April 2023 - December 2023
- Leadership
- Raphaela Erbel
Sarah Dreher
Prof. Dr. Philipp Brune
AL-KO Geräte GmbH (opens in a new window)
- Status
- Completed
Project description
This cooperation project with AL-KO Geräte GmbH (opens in a new window) focuses on the development, evaluation and quantification of AI-supported demand planning for spare parts in the garden tool industry. The historical sales data of our practice partner formed the basis of the demand planning in this time series analysis. The main objective of the application is to minimize delivery times to the end customer despite pronounced seasonal fluctuations and thus ensure an efficient and fast supply of the required components to the customer.
Scientifically, the project focuses on the question of which specific variables have a significant influence on the demand planning of spare parts. We are investigating how the use of AI methods can improve the demand planning process and what data quality and quantity is required for this. Classic AI methods such as SARIMA (Seasonal Autoregressive Integrated Moving Average) and VAR (Vector Autoregression) as well as modern deep learning techniques such as LSTM (Long Short-Term Memory) are analysed and compared for their suitability and efficiency in this specific context.
While our main focus is on the garden tool industry, the insights and experience gained are also transferable to other industries. This provides us with valuable insights into the practical application of AI methods in time series analysis, which in turn form the basis for further research and project work.