Lying until the beams bend - and in the service of science? This is the chance for test subjects willing to fib in the research project VACE (Voice Analysis for Customer Emotions), which the Center for Research on Service Sciences (CROSS) at HNU is currently conducting together with the Technology Transfer Centre Günzburg (TTZ). Scientists there are developing, training and testing an artificial intelligence (AI) that detects the smallest changes in human speech.
[1]
Human speech: an exciting field of research
Hardly any human performance is as complicated as that of speaking. Initiated by neuronal signals and processes in the brain, more than 100 muscles and several organs throughout the body are involved - for example, in addition to the larynx, lips and nose, the diaphragm comes into play as soon as we raise our voice. Body tension also plays a decisive role. In view of this complex interplay of motor and cognitive processes, it is obvious that a person's voice reveals a lot about his or her physical and mental condition. For Prof. Dr. Heiko Gewald (opens in a new window), Fabian Thaler (opens in a new window), and Prof. Dr. Stefan Fausser (opens in a new window)at the Institute for Service Management at HNU (CROSS), language and the human voice are therefore one thing above all: a highly interesting field of research.
Voice Analysis for Customer Emotions (VACE) is dedicated to a technically supported analysis of inner beliefs in spoken language. The focus is on capturing and interpreting patterns from the voice of potential customers in order to offer companies a way to optimise individual customer behaviour.
[2]
Aim of the project: filtering out the "inner conviction" at the auditory level.
The scientists are primarily concerned with the great potential offered by voice analysis through AI: Whether speech-based coronation tests or personality tests in recruiting processes, AI-supported speech and voice analyses are being used more and more. Gewald ,Thaler, and Fausser are dedicated to the technically supported analysis of beliefs in spoken language. With the VACE project, the scientists are pursuing an overarching goal in two different sub-projects and data sets: they want to extract the "inner conviction" of speakers at the auditory level and thereby operationalise actual intentions. The markers identified in this way will be used in the VACE project to develop optimised marketing strategies. The more precisely the needs, desires and intentions of customers are sounded out, the more individually individualised the response to individual needs can be, and ultimately not only can customer satisfaction be increased, but the marketing budget can also be used in a more targeted manner.
To this end, the researchers are teaching a prototypically developed AI to recognise the most subtle modifications in the voice - in principle a procedure similar to the lie detector. However, the latter includes other parameters in its analysis, such as skin conductivity, blood pressure and pulse, whereas the VACE studies focus purely on the acoustics of the spoken word.
Test persons wanted
Curious? If you would like to be a test person and oscillate between fiction and truth in the service of science, or if you would like to work on the project as a student assistant, you can contact Fabian Thaler directly by email.
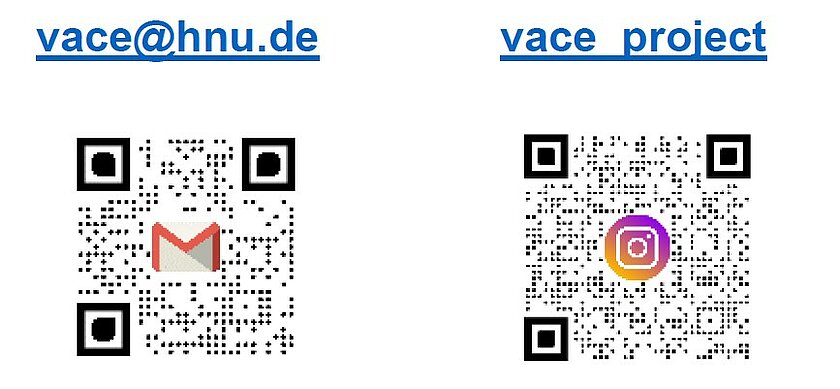
TTZ Günzburg
The Technology Transfer Centre Big Data based Marketing (TTZ Günzburg) (opens in a new window), supported by the Free State of Bavaria, was founded in 2020 as an institute of the University of Applied Sciences Neu-Ulm (HNU) in the Swabian town of Günzburg and conducts applied research and development in the field of data-driven marketing.
The interdisciplinary team advises companies strategically and operationally on the development and implementation of prototypes and concepts for the use of artificial intelligence in marketing.
[3]
Hula-hoop on Mount Everest - or: it's all about setting the right tone
The researchers are not interested in direct communication between man and machine or in extracting meaning from machine-recorded speech; that falls into the field of application of so-called Natural Language Processing (NLP). In their experiments, it is much more imperative to let the semantic level take a back seat to the acoustic level - what is important is not what is spoken, but how. "If I tell you, for example, that I danced the hula hoop naked on Mount Everest last week, you know immediately that I am just pulling your leg," says Fabian Thaler. "The algorithm doesn't understand that. But it can, and that's the interesting thing for us, recognise purely on an acoustic level that something must be wrong with the story - for example, because my pitch changes or my voice trembles." The AI uses voice samples for comparison: The algorithm learns to detect changes in pitch, speech tempo or intonation in front of a comparison foil that is collected in advance.
[4]
"It's on the tip of my tongue": Early Alzheimer's detection through voice analysis
In the long term, this research should serve another purpose besides optimising marketing strategies: early Alzheimer's detection. Even in the early stages, the most subtle nuances in speech production can indicate the presence of this degenerative disease. Even before clearly recognisable and audible speech and communication disorders or aphasia, the complete loss of speech, word-finding problems and syntactic or semantic deficits, for example, are symptoms whose early recognition enables intervention. The analysis of language can therefore help to detect this disorder earlier - for example, within the framework of apps that provide for regular language tests and thus allow an individual measurement of language.
The research at CROSS closes a research gap. While corresponding data sets have already been generated in the international context, no equivalent studies are yet available for the German-speaking world, as Fabian Thaler explains. However, because possible markers can differ greatly from language to language and from culture to culture, and the international results are therefore not transferable without compromises, a German corpus is absolutely necessary.
Our interlocutor
Fabian Thaler
is a research assistant in the field of AI / Speech Recognition at the Center for Research on Service Sciences (CROSS). After his Bachelor's degree in Business Informatics, he completed the Master's degree in Business Intelligence and Business Analytics at HNU. In his master's thesis, he proved that machine learning techniques can be used to derive recommendations for action for a potential Alzheimer's disease and is currently continuing research in this area as part of a dissertation project.
When I'm not doing research/work, I ...
... prefer to spend my time with my family and/or friends (ideally at a concert).
Current reading:
Dr. Leon Windscheid: Feeling Better - A Journey to Serenity.
My area of expertise in a few words:
Making the most of AI
My next publication will be ...
... deal with the possibilities of recognising/classifying the truth content of narratives (speech) on a purely acoustic level with the help of artificial intelligence.
Scientific work/promotion is ...
... a very exciting and multi-layered activity for me personally, where you are challenged in a different way every day and can thus not only advance your own field of research, but also constantly reinvent and develop yourself.
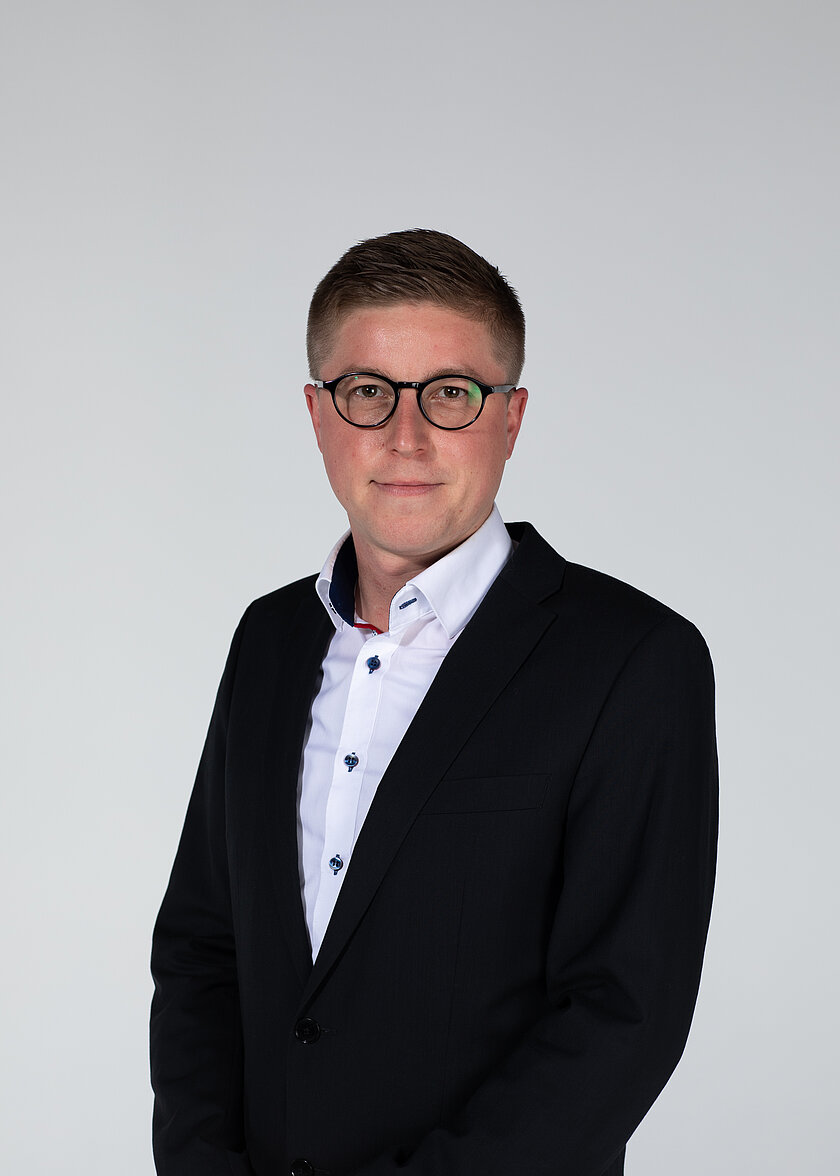
Natural language is the interface of the future
[5]
Data, data, data: voice sample fodder for the algorithm
"The first and most important task is, of course, to create a suitable database," says Fabian Thaler. The more voice samples there are, the better the prototype can be trained to identify any deviations in the audio streams. The CROSS team is collecting the corresponding samples in two different studies.
The researchers had already conducted the study of the first sub-project, the debating club. The aim was to find out whether verbal statements that do not correspond to the actual opinion of the speakers show discrepancies in the acoustic and linguistic features. The algorithm worked successfully: it was able to identify the "inner conviction" of the speakers with an accuracy of around 98 percent. However, because the study did not have enough participants, the project is now going into a third round. The test persons receive a randomly selected, polarising topic by email, 30 minutes of preparation time and a specific role assignment; they are therefore only told shortly beforehand whether they are to take a pro or con stance on the said question and argue accordingly.
In the second sub-project, the participants are allowed to be even more creative: They present the researchers with five previously prepared short stories, at least one of which must be invented. "There were some extremely imaginative narratives that we in the evaluation team were able to identify relatively unanimously as lies," Fabian Thaler reports from the samples so far. "The exciting thing now is to find out whether the AI can also identify this as a lie, regardless of the abstruse content."
[6]
From spectrum to cepstrum
To do this, however, the audio data must first be fed in. Fabian Thaler shows us how this works directly in the system. The first step in automatic speech recognition is to extract certain features from the digital audio signal. "The classic spectrum, as everyone knows it from audio recordings, is too inaccurate for our analysis," he explains. "We have to turn the whole thing around again, so to speak, and approximate the human voice." This is called cepstrum - an anagram of spectrum. So-called MFCCs (Mel Frequency Cepstral Coefficients), which are filtered out in the process. These allow conclusions to be drawn about a variety of acoustic speech features.
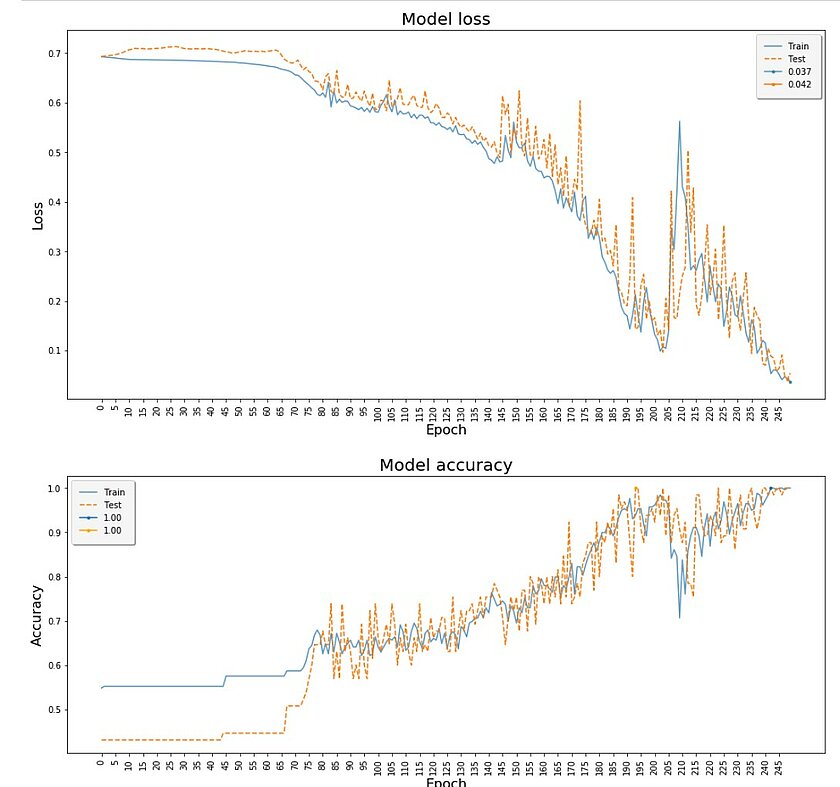
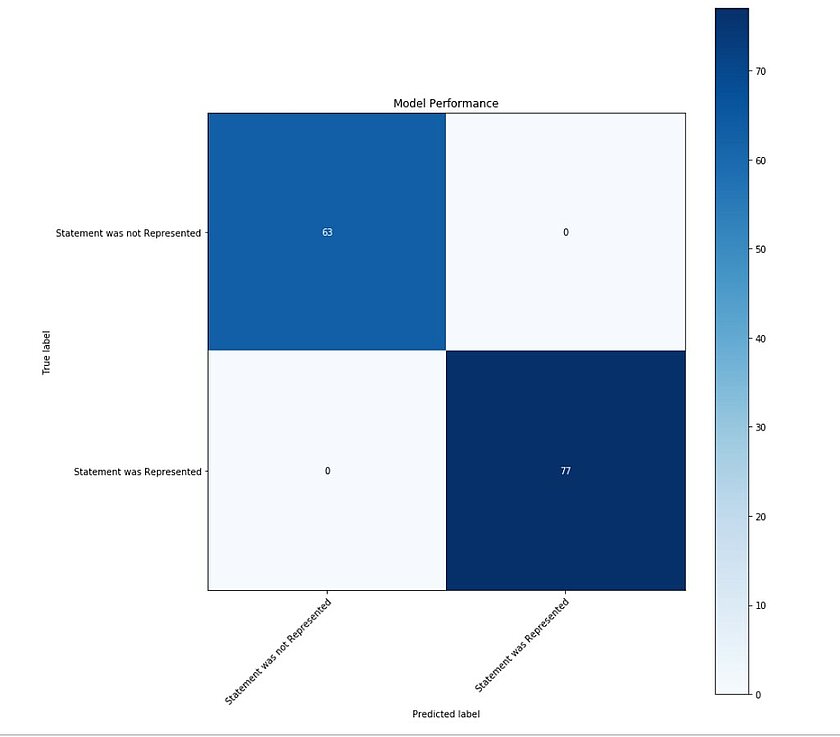
[7]
Quo vadis, voice analysis
Automated natural language processing is on the rise and opens up manifold possibilities in almost all areas of our lives. Speech recognition systems are getting better and better at understanding human speech - but an AI is only as good as the data it is provided with. In the coming weeks and months, Fabian Thaler will therefore have many more fairy tale lessons on his calendar: to ensure a sufficient database, many more voice samples will be collected from volunteers, fed in and measured. We will continue to accompany him and the project and will publish regular updates here.
What actually is ... ?
Speech Recognition
is an interdisciplinary subfield of computer science and computational linguistics. It focuses on the development of methods and technologies that enable computers to recognise and translate spoken language into text.
[Translate to English:] Machine Learning
is an area of artificial intelligence. Machine learning techniques allow IT systems to recognise patterns in data sets from which solutions can be developed.
Blathering, lying, sweating
Die VACE-Experimente im Praxistest
A little fibbing can't be that difficult, I think to myself as I read up on the subject on the Internet. A quick, unscientific Google search reveals that people lie up to 200 times a day, mostly unconsciously. So it should be child's play to come up with a few outrageous stories for a good research purpose. But before I'm allowed to present myself as Captain Blue Bear, I'll first start as a test person for the first research project: the debating club. Here I don't have to invent my own fables, but if necessary I have to argue against my own attitude - the very "inner conviction" that the VACE team wants to detect auditorily. Of course, Fabian Thaler cannot give me too much information in advance, after all, I should approach the matter with as little bias as possible.
I eagerly open the invitation email, hoping for an interesting topic - and bang on the money: I am to argue for the reintroduction of the death penalty in Germany, including public executions. I can immediately think of countless counter-arguments that I could present with flaming fervour, but to take a favourable stance that the algorithm might even take away from me...? This will be a challenge, especially since my acting career was already crowned with moderate success at best in the middle school theatre group. I am tempted to google up a few pro-arguments - but I was forbidden to do so beforehand, and as an exemplary test person I will of course follow all instructions. The fact that only the audio stream is recorded at least suits me, so I don't have to worry about my heated cheeks or a nervous twitching of the eyes that might indicate possible fibbing.
After a short introduction via Zoom, the show starts immediately: picture off, sound on, the stage is mine. I hastily drink a sip of water, clear my throat and begin my plea for the reintroduction of the death penalty, including public executions. With great difficulty I present the arguments I have scraped together, gape and gape, and involuntarily pepper my speech with a number of umms and hmmms.
"You spoke relatively quickly," Fabian Thaler explains to me in the subsequent discussion, "that might be an indicator for the AI that the statement and the inner attitude do not match. I explain to him that I generally tend to talk fast - can the AI distinguish that? It can't, explains the scientist, but it does take this into account: a questionnaire that you fill out in advance helps the researchers in the subsequent evaluation to adjust the analysis for factors such as nervousness or other dispositions. Over the next few weeks, the AI will be fed my audio stream and my "inner conviction" will be put through its paces. Exciting!
While participation in the debating club was still compulsory for liars willing to learn, now comes the freestyle. For the second project, I have to dig deeper into my imagination: I have to prepare and present five one-minute stories. What exactly I tell the researchers is up to me. Whether it's stories from the last holiday, childhood memories or experiences from everyday life at work, the only important thing is that at least one of my stories is a lie. If I manage to outsmart the scientists - either by selling them credible lies as true or true stories as fictitious - my chances of being rewarded increase. So it goes without saying that I prepare my stories meticulously. "He who lies once is not believed"? Let's see... Because the CROSS team is still looking for more test subjects for this study, I can't reveal too much at this point - just this: I didn't become a Baroness Münchhausen in the second experiment either.
Read on
If you want to read more about artificial intelligence, Fabian Thaler recommends "Macht Euch die Maschinen untertan. On Dealing with Artificial Intelligence" by Andrian Kreye.